2022.07.11 コラム
GTM × GA4 × BigQuery|サーバーサイドCookie単位でUB集計してみた
● はじめに
本記事は、マーケティング業務に従事している方であれば一度は聞いた事や
触れた事のあるGoogleAnalytics(GA)とGoogleBigQueryを活用した
データ分析事例についてご紹介しております。
DataCurrentでは、クッキーレス対策のひとつとして、Googleソリューションを活用したマーケティング基盤の構築支援を提供しています。クッキーレスに対応したデータ取得方法のみならず、そのデータを活用した機械学習、ダッシュボード開発、分析支援などをサポートします。
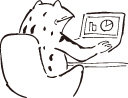
本記事では、分析支援についてご紹介致します。
● こんな方におすすめ
- 自社のデータだけでも深掘りできる環境を持ちたい
- 管理画面だけでは施策に活かしづらい
- データを活用して機械学習を実施したい
● 従来とGA4の違い
① BigQueryの連携が可能
BigQueryとはGoogle Cloud Platform 内で提供されるサービスで、
大規模なクエリによるデータ解析をすることが出来る、データウェアハウスのことを指します。
BigQueryとの連携はこれまでのユニバーサルアナリティクス(UA)の場合、有料版のGA360の契約をする必要がありました。
しかしGA4では無料アカウントでもBigQueryと連携することが出来、これまで以上に大規模かつ柔軟な分析が可能になりました。
②計測方法の変化
GA4では基本的にイベントトラッキングによりデータを収集しているため、
UAで取得しているPV(ページビュー)のような概念が無くなります。
これまでアプリのデータをFirebase等で見てきたユーザーであれば問題ないかもしれませんが、
ウェブのデータをUAで見てきたユーザーだと最初は慣れないかもしれません。
※ユーザーが起こしたアクションをイベントとして(CVとして)計測していくことになります。
● 今回の分析目的
- 目的
閲覧ユーザー数が多いコンテンツをサーバーサイドcookieで確認したい! - 背景
管理画面では集計後の結果のみの可視化であるため
GA4のログがたまったBQを使用して、様々な分析をしてみる。
●実際の分析手順
早速BQで集計開始・・・・!
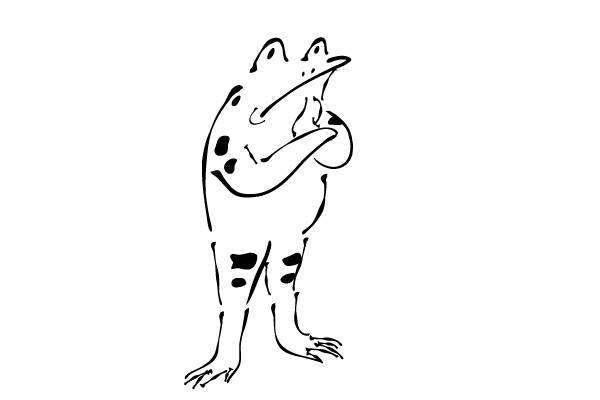
IDとUBを集計するだけ?すごく簡単そうに見えるが
実際のSQLクエリってどう書いているの?
- GA4のログを取得する際に、必要なSQL文は下記の通り
SELECT FORMAT_TIMESTAMP('%Y-%m-%d',(TIMESTAMP_MICROS(event_timestamp)), 'Asia/Tokyo') AS event_timestamp_format, user_pseudo_id, event_date, event_timestamp, event_name, (select case when p.value.int_value is not null then safe_cast(p.value.int_value as string) else null end from unnest(event_params) p where p.key = "ga_session_id") ga_session_id, (select case when p.value.int_value is not null then safe_cast(p.value.int_value as string) else null end from unnest(event_params) p where p.key = "engaged_session_event") engaged_session_event, (select case when p.value.int_value is not null then safe_cast(p.value.int_value as string) else null end from unnest(event_params) p where p.key = "ga_session_number") ga_session_number, (select case when p.value.string_value is not null then safe_cast(p.value.string_value as string) else null end from unnest(event_params) p where p.key = "page_referrer") page_referrer, (select case when p.value.string_value is not null then safe_cast(p.value.string_value as string) else null end from unnest(event_params) p where p.key = "page_location") page_location, (select case when p.value.string_value is not null then safe_cast(p.value.string_value as string) else null end from unnest(event_params) p where p.key = "page_title") page_title, event_previous_timestamp, event_value_in_usd, event_bundle_sequence_id, event_server_timestamp_offset, user_id, user_first_touch_timestamp, user_ltv.revenue AS user_ltv_revenue, user_ltv.currency AS user_ltv_currency, device.category AS device_category, device.mobile_brand_name AS device_mobile_brand_name, device.mobile_model_name AS device_mobile_model_name, device.mobile_marketing_name AS device_mobile_marketing_name, device.mobile_os_hardware_model AS device_mobile_os_hardware_model, device.operating_system AS device_operating_system, device.operating_system_version AS device_operating_system_version, device.vendor_id AS device_vendor_id, device.advertising_id AS device_advertising_id, device.language AS device_language, device.time_zone_offset_seconds AS device_time_zone_offset_seconds, device.is_limited_ad_tracking AS device_is_limited_ad_tracking, device.browser AS device_browser, device.browser_version AS device_browser_version, device.web_info.browser AS device_web_info_browser, device.web_info.browser_version AS device_web_info_browser_version, device.web_info.hostname AS device_web_info_hostname, geo.continent AS geo_continent, geo.sub_continent AS geo_sub_continent, geo.country AS geo_country, geo.region AS geo_region, geo.metro AS geo_metro, geo.city AS geo_city, traffic_source.name AS traffic_source_name, traffic_source.medium AS traffic_source_medium, traffic_source.source AS traffic_source_source, stream_id, platform, event_dimensions.hostname AS event_dimensions_hostname, ecommerce.total_item_quantity AS ecommerce_total_item_quantity, ecommerce.purchase_revenue_in_usd AS ecommerce_purchase_revenue_in_usd, ecommerce.purchase_revenue AS ecommerce_purchase_revenue, ecommerce.refund_value_in_usd AS ecommerce_refund_value_in_usd, ecommerce.refund_value AS ecommerce_refund_value, ecommerce.shipping_value_in_usd AS ecommerce_shipping_value_in_usd, ecommerce.shipping_value AS ecommerce_shipping_value, ecommerce.tax_value_in_usd AS ecommerce_tax_value_in_usd, ecommerce.tax_value AS ecommerce_tax_value, ecommerce.unique_items AS ecommerce_unique_items, ecommerce.transaction_id AS ecommerce_transaction_id, (SELECT item_id FROM UNNEST(items)) AS item_id, (SELECT item_name FROM UNNEST(items)) AS item_name, (SELECT item_brand FROM UNNEST(items)) AS item_brand, (SELECT item_variant FROM UNNEST(items)) AS item_variant, (SELECT item_category FROM UNNEST(items)) AS item_category, (SELECT item_category2 FROM UNNEST(items)) AS item_category2, (SELECT item_category3 FROM UNNEST(items)) AS item_category3, (SELECT item_category4 FROM UNNEST(items)) AS item_category4, (SELECT item_category5 FROM UNNEST(items)) AS item_category5, (SELECT price_in_usd FROM UNNEST(items)) AS price_in_usd, (SELECT Price FROM UNNEST(items)) AS price, (SELECT quantity FROM UNNEST(items)) AS quantity, (SELECT item_revenue_in_usd FROM UNNEST(items)) AS item_revenue_in_usd, (SELECT item_revenue FROM UNNEST(items)) AS item_revenue, (SELECT item_refund_in_usd FROM UNNEST(items)) AS item_refund_in_usd, (SELECT item_refund FROM UNNEST(items)) AS item_refund, (SELECT coupon FROM UNNEST(items)) AS coupon, (SELECT affiliation FROM UNNEST(items)) AS affiliation, (SELECT location_id FROM UNNEST(items)) AS location_id, (SELECT item_list_id FROM UNNEST(items)) AS item_list_id, (SELECT item_list_name FROM UNNEST(items)) AS item_list_name, (SELECT item_list_index FROM UNNEST(items)) AS item_list_index, (SELECT promotion_id FROM UNNEST(items)) AS promotion_id, (SELECT promotion_name FROM UNNEST(items)) AS promotion_name, (SELECT creative_name FROM UNNEST(items)) AS creative_name, (SELECT creative_slot FROM UNNEST(items)) AS creative_slot FROM `table`
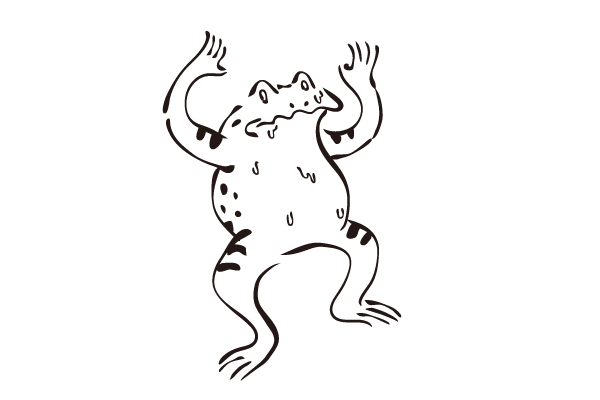
なんと!分析対象を抜き出すだけでも難しそう。。。
これに加えてURL毎の人数を集計するSQLを追加か、、、大変だ!
● データの可視化
先ほどのSQLを用いて、URLの抽出とサーバーサイドcookie単位で集計してみました。
BigQueryで出力される結果は下記のとおりです。
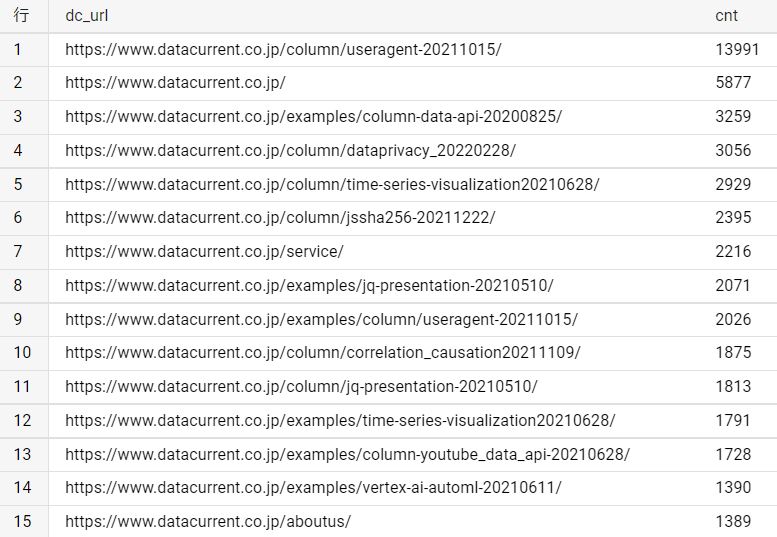
また、ダッシュボードにより、可視化/一元管理することも可能です。
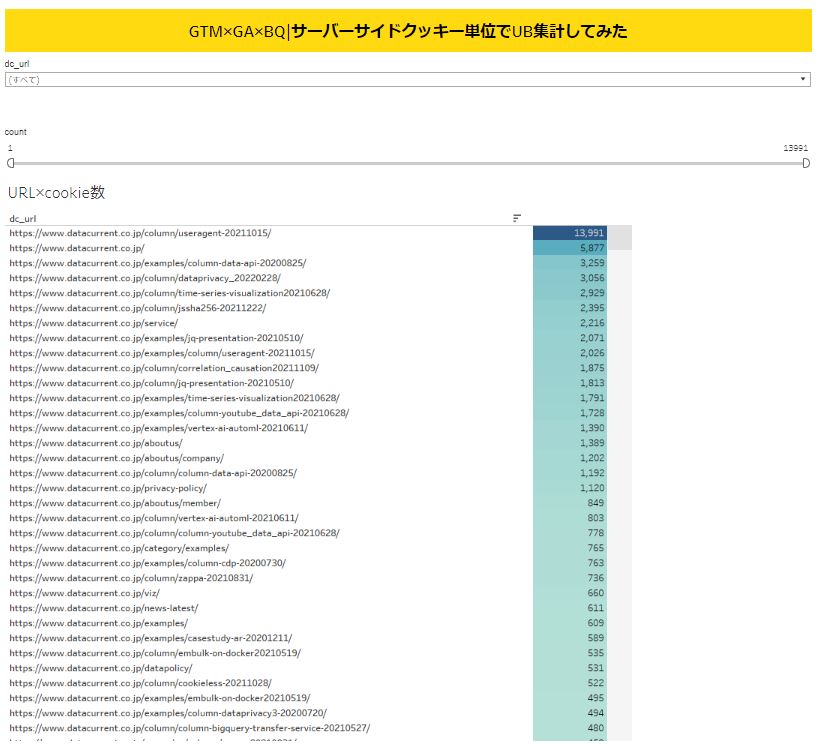
● ご紹介まとめ
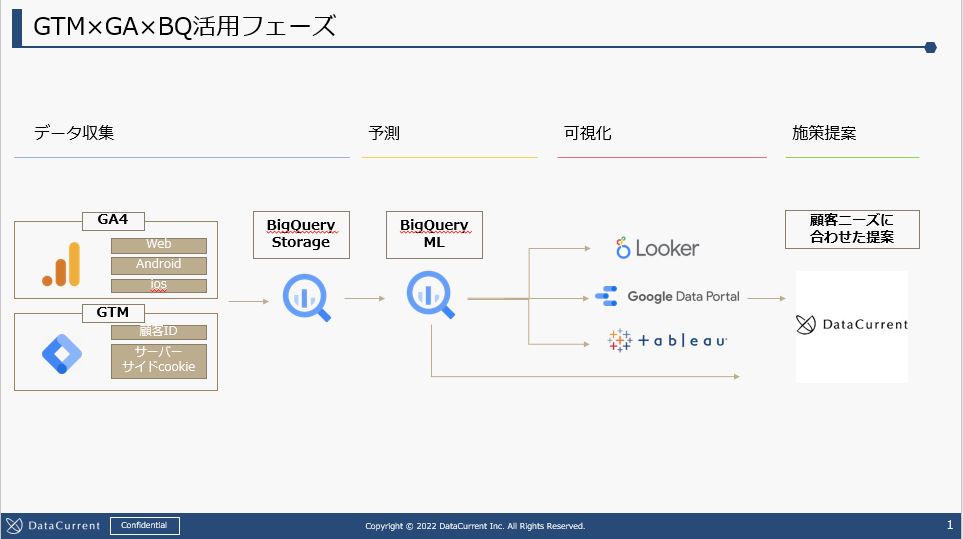
■本日ご紹介した事例の関連サポート
・GA4導入サポート
・GTMタグ設置サポート
・BigQueryエクスポート設定サポート
・BigQueryのデータを活用したSQL分析サポート
・BigQuery機械学習サポート
※BQ以外にも、弊社自社開発機械学習ツールなども活用可能
・BIツールを用いた可視化サポート
・GCP認定パートナー、リセール可能
⇒ニーズに合わせた分析と可視化、施策提案までサポート可能
その他にも、下記にてGA4についてやGA4関連サービスをご紹介しているので是非ご覧ください!
▼GA関連コラム記事
⇒Googleアナリティクス4(GA4)とは?導入とそのポイントについて<前編>
⇒Googleアナリティクス4(GA4)とは?移行とそのポイントについて<後編>
▼GA関連サービス
》「GA4×Google Cloud×GTMを活用したクッキーレス対策」の詳細はこちら
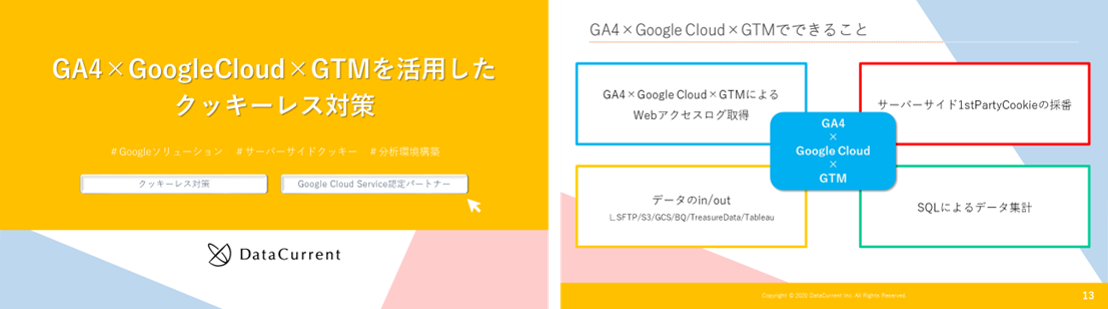
※DC社では、他にもGA4の導入、導入後の活用施策、データを活用した機械学習をご提案させていただいております。ご興味がある方は下記フォームにてお問合せいただければと思います。
本件に関するお問い合わせは下記にて承ります。
株式会社DataCurrent
info@datacurrent.co.jp